By Shelby Hiter
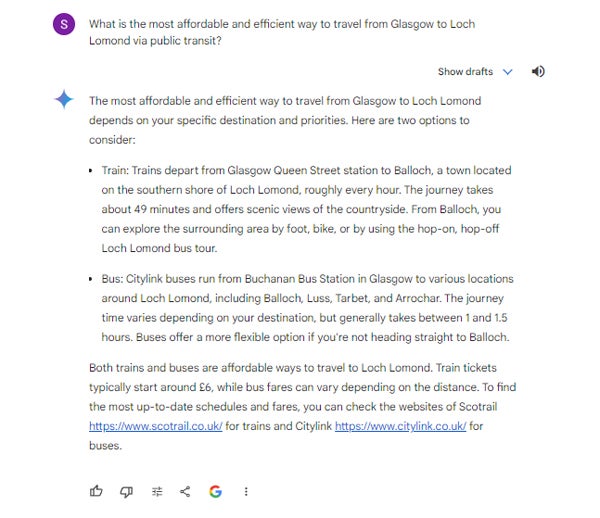
Impact of AI on Privacy Laws and Regulations
Most privacy laws and regulations do not yet directly address AI and how it can be used or how data can be used in AI models. As a result, AI companies have had a lot of freedom to do what they want. This has led to ethical dilemmas like stolen IP, deepfakes, sensitive data exposed in breaches or training datasets, and AI models that seem to act on hidden biases or malicious intent.
More regulatory bodies—both governmental and industry-specific—are recognizing the threat AI poses and developing privacy laws and regulations that directly address AI issues. Expect more regional, industry-specific, and company-specific regulations to come into play in the coming months and years, with many of them following the EU AI Act as a blueprint for how to protect consumer privacy.
Public Perception and Awareness of AI Privacy Issues
Since ChatGPT was released, the general public has developed a basic knowledge of and interest in AI technologies. Despite the excitement, general public perception of AI technology is fearful—especially as it relates to AI privacy.
Many consumers do not trust the motivations of big AI and tech companies and worry that their personal data and privacy will be compromised by the technology. Frequent mergers, acquisitions, and partnerships in this space can lead to emerging monopolies, and the fear of the power these organizations have.
According to a survey completed by the International Association of Privacy Professionals in 2023, 57 percent of consumers fear that AI is a significant threat to their privacy, while 27 percent felt neutral about AI and privacy issues. Only 12 percent disagreed that AI will significantly harm their personal privacy.
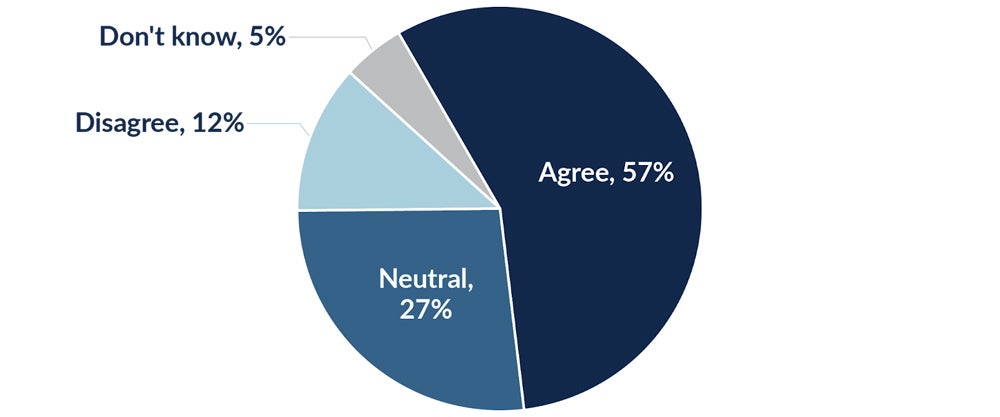
AI privacy concerns are growing as emerging technologies like generative AI become more integrated into daily life. Business leaders dealing with AI and privacy issues must understand the technology’s great potential even as they guard against the accompanying privacy and ethical issues.
This guide explores some of the most common AI and privacy concerns that businesses face. Additionally, it identifies potential solutions and best practices organizations can pursue to achieve better outcomes for their customers, their reputation, and their bottom line.
KEY TAKEAWAYS
- •Customers demand data privacy, but some popular AI technologies collect and use personal data in unauthorized or unethical ways.
- •AI is currently a largely unregulated business technology, which leads to a variety of privacy concerns; more regulations are expected to pass into law in the future.
- •Your business’s strict adherence to data, security, and regulatory best practices can protect your customers against AI privacy issues.
- •Ultimately, it is a business leader’s responsibility to hold both their chosen AI vendors and their employees accountable for AI and data privacy.
- •While many AI vendors are working to improve their models’ transparency and approach to personal data, business users need to scour their current policies and strategies before inputting sensitive data into any third-party system.
TABLE OF CONTENTS
Major Issues with AI and Privacy
Given that the role of artificial intelligence has grown so rapidly, it’s not surprising that issues like unauthorized incorporation of user data, unclear data policies, and limited regulatory safeguards have created significant issues with AI and privacy.
Unauthorized Incorporation of User Data
When users of AI models input their own data in the form of queries, there’s the possibility that this data will become part of the model’s future training dataset. When this happens, this data can show up as outputs for other users’ queries, which is a particularly big issue if users have input sensitive data into the system.
In a now-famous example, three different Samsung employees leaked sensitive company information to ChatGPT that could now possibly be part of ChatGPT’s training data. Many vendors, including OpenAI, are cracking down on how user inputs are incorporated into future training. But there’s still no guarantee that sensitive data will remain secure and outside of future training sets.
Unregulated Usage of Biometric Data
A growing number of personal devices use facial recognition, fingerprints, voice recognition, and other biometric data security instead of more traditional forms of identity verification. Public surveillance devices are also beginning to use AI to scan for biometric data so individuals can be identified quickly.
While these new biometric security tools are incredibly convenient, there’s limited regulation focused on how AI companies can use this data once it’s collected. In many cases, individuals don’t even know that their biometric data has been collected, much less that it is being stored and used for other purposes.
Covert Metadata Collection Practices
When a user interacts with an ad, a TikTok or other social media video, or pretty much any web property, metadata from that interaction—as well as the person’s search history and interests—can be stored for more precise content targeting in the future.
This method of metadata collection has been going on for years, but with the help of AI, more of that data can be collected and interpreted at scale, making it possible for tech companies to further target their messages at users without their knowledge of how it works. While most user sites have policies that mention these data collection practices and/or require users to opt in, it’s mentioned only briefly and in the midst of other policy text, so most users don’t realize what they’ve agreed to. This veiled metadata collection agreement subjects users and everything on their mobile devices to scrutiny.
Limited Built-In Security Features for AI Models
While some AI vendors may choose to build baseline cybersecurity features and protections into their models, many AI models do not have native cybersecurity safeguards in place. Even the AI technologies that do have basic safeguards rarely come with comprehensive cybersecurity protections. This is because taking the time to create a safer and more secure model can cost AI developers significantly, both in time to market and overall development budget.
Whatever the reason, AI developers’ limited focus on security and data protection makes it much easier for unauthorized users and bad-faith actors to access and use other users’ data, including personally identifiable information (PII).
Extended and Unclear Data Storage Policies
Few AI vendors are transparent about how long, where, and why they store user data. The vendors who are often store data for lengthy periods of time, or use it in ways that clearly do not prioritize privacy.
For example, OpenAI’s privacy policy says it can “provide Personal Information to vendors and service providers, including providers of hosting services, customer service vendors, cloud services, email communication software, web analytics services, and other information technology providers, among others. Pursuant to our instructions, these parties will access, process, or store Personal Information only in the course of performing their duties to us.”
In this case, several types of companies can gain access to your ChatGPT data for various reasons as determined by OpenAI. It’s especially concerning that “among others” is a category of vendors that can collect and store your data, as there’s no information about what these vendors do or how they might choose to use or store your data.
OpenAI’s policy provides additional information about what data is typically stored and what your privacy rights are as a consumer. You can access your data and review some information about how it’s processed, delete your data from OpenAI records, restrict or withdraw information processing consent, and/or submit a formal complaint to OpenAI or local data protection authorities.
This more comprehensive approach to data privacy is a step in the right direction, but the policy still contains certain opacities and concerning elements, especially for Free and Plus plan users who have limited control over or visibility into how their data is used.
Little Regard for Copyright and IP Laws
AI models pull training data from all corners of the web. Unfortunately, many AI vendors either don’t realize or don’t care when they use someone else’s copyrighted artwork, content, or other intellectual property without their consent.
Major legal battles have focused on AI image generation vendors like Stability AI, Midjourney, DeviantArt, and Runway AI. It is alleged that several of these tools scraped artists’ copyrighted images from the internet without permission. Some of the vendors defended their action by citing a lack of laws that prevent them from following this process for AI training.
The problems of using unauthorized copyrighted products and IP grow much worse as AI models are trained, retrained, and fine-tuned with this data over time. Many of today’s AI models are so complex that even their builders can’t confidently say what data is being used, where it came from, and who has access to it.
Limited Regulatory Safeguards
Some countries and regulatory bodies are working on AI regulations and safe use policies, but no overarching standards are officially in place to hold AI vendors accountable for how they build and use artificial intelligence tools. The proposed regulation closest to becoming law is the EU AI Act, expected to be published in the Official Journal of the European Union in summer of 2024. Some aspects of the law will take as long as three years to become enforceable.
With such limited regulation, a number of AI vendors have come under fire for IP violations and opaque training and data collection processes, but little has come from these allegations. In most cases, AI vendors decide their own data storage, cybersecurity, and user rules without interference.
How Data Collection Creates AI Privacy Issues
Unfortunately, the total number and variety of ways that data is collected all but ensures that this data will find its way into some irresponsible uses. From Web scraping to biometric technology to IoT sensors, modern life is essentially lived in service of data collection efforts.
Web Scraping Harvests a Wide Net
Because web scraping and crawling require no special permissions and enable vendors to collect massive amounts of varied data, AI tools often rely on these practices to quickly build training datasets at scale. Content is scraped from publicly available sources on the internet, including third-party websites, wikis, digital libraries, and more. In recent years, user metadata is also increasingly pulled from marketing and advertising datasets and websites with data about targeted audiences and what they engage with most.
User Queries in AI Models Retain Data
When a user inputs a question or other data into an AI model, most AI models store that data for at least a few days. While that data may never be used for anything else, many artificial intelligence tools collect that data and hold onto it for future training purposes.
Biometric Technology Can Be Intrusive
Surveillance equipment—including security cameras, facial and fingerprint scanners, and microphones—can all be used to collect biometric data and identify humans without their knowledge or consent. State by state, rules are getting stricter in the U.S. regarding how transparent companies need to be when using this kind of technology. However, for the most part, they can collect this data, store it, and use it without asking customers for permission.
IoT Sensors and Devices Are Always On
Internet of Things (IoT) sensors and edge computing systems collect massive amounts of moment-by-moment data and process that data nearby to complete larger and quicker computational tasks. AI software often takes advantage of an IoT system’s detailed database and collects its relevant data through methods like data learning, data ingestion, secure IoT protocols and gateways, and APIs.
APIs Interface With Many Applications
APIs give users an interface with different kinds of business software so they can easily collect and integrate different kinds of data for AI analysis and training. With the right API and setup, users can collect data from CRMs, databases, data warehouses, and both cloud-based and on-premises systems. Given how few users pay attention to the data storage and use policies their software platforms follow, it’s likely many users have had their data collected and applied to different AI use cases without their knowledge.
Public Records Are Easy Accessed
Whether records are digitized or not, public records are often collected and incorporated into AI training sets. Information about public companies, current and historical events, criminal and immigration records, and other public information can be collected with no prior authorization required.
User Surveys Drives Personalization
Though this data collection method is more old-fashioned, using surveys and questionnaires are still a tried-and-true way that AI vendors collect data from their users. Users may answer questions about what content they’re most interested in, what they need help with, how their most recent experience with a product or service was, or any other question that gives the AI a better idea about how to personalize interactions.
Emerging Trends in AI and Privacy
Because the AI landscape is evolving so rapidly, the emerging trends shaping AI and privacy issues are also changing at a remarkable pace. Among the leading trends are major advances in AI technology itself, the rise of regulations, and the role of public opinion on AI’s growth.
Advancements in AI Technologies
AI technologies have exploded in terms of technology sophistication, use cases, and public interest and knowledge. This growth has happened with more traditional AI and machine learning technologies but also with generative AI.
Generative AI’s large language models (LLMs) and other massive-scale AI technologies are trained on incredibly large datasets, including internet data and some more private or proprietary datasets. While the data collection and training methodologies have improved, AI vendors and their models often are not transparent in their training or the algorithmic processes they use to generate answers.
To address this issue, many generative AI companies in particular have updated their privacy policies and their data collection and storage standards. Others, such as Anthropic and Google, have worked to develop and release clear research that illustrates how they are working to incorporate more explainable AI practices into their AI models, which improves transparency and ethical data usage across the board.
Impact of AI on Privacy Laws and Regulations
Most privacy laws and regulations do not yet directly address AI and how it can be used or how data can be used in AI models. As a result, AI companies have had a lot of freedom to do what they want. This has led to ethical dilemmas like stolen IP, deepfakes, sensitive data exposed in breaches or training datasets, and AI models that seem to act on hidden biases or malicious intent.
More regulatory bodies—both governmental and industry-specific—are recognizing the threat AI poses and developing privacy laws and regulations that directly address AI issues. Expect more regional, industry-specific, and company-specific regulations to come into play in the coming months and years, with many of them following the EU AI Act as a blueprint for how to protect consumer privacy.
Public Perception and Awareness of AI Privacy Issues
Since ChatGPT was released, the general public has developed a basic knowledge of and interest in AI technologies. Despite the excitement, general public perception of AI technology is fearful—especially as it relates to AI privacy.
Many consumers do not trust the motivations of big AI and tech companies and worry that their personal data and privacy will be compromised by the technology. Frequent mergers, acquisitions, and partnerships in this space can lead to emerging monopolies, and the fear of the power these organizations have.
According to a survey completed by the International Association of Privacy Professionals in 2023, 57 percent of consumers fear that AI is a significant threat to their privacy, while 27 percent felt neutral about AI and privacy issues. Only 12 percent disagreed that AI will significantly harm their personal privacy.
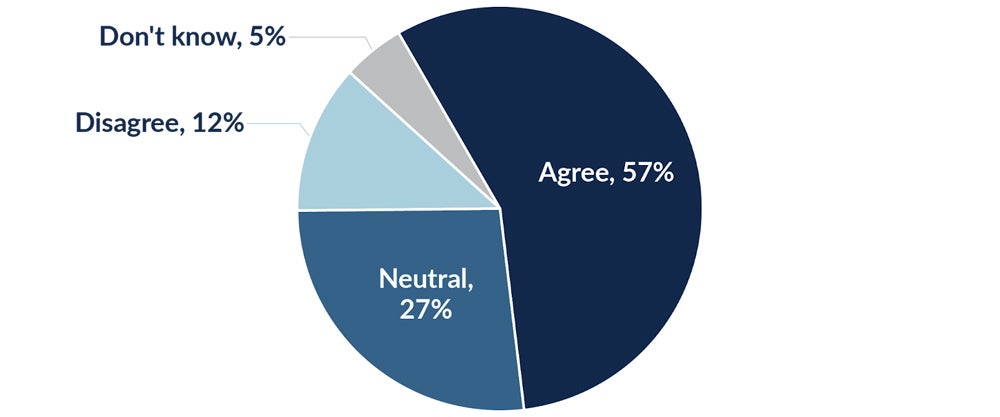
Real-World Examples of AI and Privacy Issues
While there have been several significant and highly publicized security breaches with AI technology and its respective data, many vendors and industries are taking important strides in the direction of better data protections. We cover both failures and success in the following examples.
High-Profile Privacy Issues Involving AI
Here are some of the most major breaches and privacy violations that directly involved AI technology over the past several years:
- Microsoft: Its recent announcement of the Recall feature, which allows business leaders to collect, save, and review user-activity screenshots from their devices, received significant pushback for its lack of privacy design elements, as well as for the company’s recent problems with security breaches. Microsoft will now let users more easily opt in or out of the process, and plans to improve data protection with just-in-time decryption and encrypted search index databases.
- OpenAI: OpenAI experienced its first major outage in March 2023 as a result of a bug that exposed certain users’ chat history data to other users, and even exposed payment and other personal information to unauthorized users for a period of time.
- Google: An ex-Google employee stole AI trade secrets and data to share with the People’s Republic of China. While this does not necessarily impact personal data privacy, the implications of AI and tech companies’ employees being able to get this kind of access are concerning.
Successful Implementations of AI with Strong Privacy Protections
Many AI companies are innovating to create privacy-by-design AI technologies that benefit both businesses and consumers, including the following:
- Anthropic: Especially with its latest Claude 3 model, Anthropic has continued to grow its constitutional AI approach, which enhances model safety and transparency. The company also follows a responsible scaling policy to regularly test and share with the public how its models are performing against biological, cyber, and other important ethicality metrics.
- MOSTLY AI: This is one of several AI vendors that has developed comprehensive technology for synthetic data generation, which protects original data from unnecessary use and exposure. The technology works especially well for responsible AI and ML development, data sharing, and testing and quality assurance.
- Glean: One of the most popular AI enterprise search solutions on the market today, Glean was designed with security and privacy at its core. Its features include zero trust security and a trust layer, user authentication, the principle of least privilege, GDPR compliance, and data encryption at rest and in transit.
- Hippocratic AI: This generative AI product, specifically designed for healthcare services, complies with HIPAA and has been received extensively by nurses, physicians, health systems, and payor partners to ensure data privacy is protected and patient data is used ethically to deliver better care.
- Simplifai: A solution for AI-supported insurance claims and document processing, Simplifai explicitly follows a privacy-by-design approach to protect its customers’ sensitive financial data. Its privacy practices include data masking, limited storage times and regular data deletion; built-in platform, network, and data security components and technology; customer-driven data deletion; data encryption; and the use of regional data centers that comply with regional expectations.
Best Practices for Managing AI and Privacy Issues
While AI presents an array of challenging privacy issues, companies can surmount these concerns by using best practices like focusing on data governance, establishing appropriate use policies and educating all stakeholders.
Invest in Data Governance and Security Tools
Some of the best solutions for protecting AI tools and the rest of your attack surface include extended detection and response (XDR), data loss prevention, and threat intelligence and monitoring software. A number of data-governance-specific tools also exist to help you protect data and ensure all data use remains in compliance with relevant regulations.
Establish an Appropriate Use Policy for AI
Internal business users should know what data they can use and how they should use it when engaging with AI tools. This is particularly important for organizations that work with sensitive customer data, like protected health information (PHI) and payment information.
Read the Fine Print
AI vendors typically offer some kind of documentation or policy that covers how their products work and the basics of how they were trained. Read this documentation carefully to identify any red flags, and if there’s something you’re not sure about or that’s unclear in their policy docs, reach out to a representative for clarification.
Use Only Non-Sensitive Data
As a general rule, do not input your business’s or customers’ most sensitive data in any AI tool, even if it’s a custom-built or fine-tuned solution that feels private. If there’s a particular use case you want to pursue that involves sensitive data, research if there’s a way to safely complete the operation with digital twins, data anonymization, or synthetic data.
Educate Stakeholders and Users on Privacy
Your organization’s stakeholders and employees should receive both general training and role-specific training for how, when, and why they can use AI technologies in their daily work. Training should be an ongoing initiative that focuses on refreshing general knowledge and incorporating information about emerging technologies and best practices.
Enhance Built-In Security Features
When developing and releasing AI models for more general use, you must put in the effort to protect user data at all stages of model lifecycle development and optimization. To improve your model’s security features, focus heavily on data, increasing practices like data masking, data anonymization, and synthetic data usage; also consider investing in more comprehensive and modern cybersecurity tool sets for protection, such as extended detection and response (XDR) software platforms.
Proactively Implement Stricter Regulatory Measures
The EU AI Act and similar overarching regulations are on the horizon, but even before these laws go into effect, AI developers and business leaders should regulate how AI models and data are used. Set and enforce clear data usage policies, provide avenues for users to share feedback and concerns, and consider how AI and its needed training data can be used without compromising industry-specific regulations or consumer expectations.
Improve Transparency in Data Usage
Increasing data usage transparency—which includes being more transparent about data sources, collection methods, and storage methods—is a good business practice all-around. It gives customers greater confidence when using your tools, it provides the necessary blueprints and information AI vendors need to pass a data or compliance audit, and it helps AI developers and vendors to create a clearer picture of what they’re doing with AI and the roadmap they plan to follow in the future.
Reduce Data Storage Periods
The longer data is stored in a third-party repository or AI model (especially one with limited security protections), the more likely that data will fall victim to a breach or bad actor. The simple act of reducing data storage periods to only the exact amount of time that’s necessary for training and quality assurance will help to protect data against unauthorized access and give consumers greater peace of mind when they discover this reduced data storage policy is in place.
Ensure Compliance with Copyright and IP Laws
While current regulations for how AI can incorporate IP and copyrighted assets are murky at best, AI vendors will improve their reputation (and be better prepared for impending regulations) if they vet their sources from the outset. Similarly, business users of AI tools should be diligent in reviewing the documentation AI vendors provide about how their data is sourced; if you have questions or concerns about IP usage, you should contact that vendor immediately or even stop using that tool.
Bottom Line: Addressing AI and Privacy Issues Is Essential
AI tools present businesses and the everyday consumer with all kinds of new conveniences, ranging from task automation and guided Q&A to product design and programming. While these tools can simplify our lives, they also run the risk of violating individual privacy in ways that can damage vendor reputation and consumer trust, cybersecurity, and regulatory compliance.
It takes extra effort to use AI in a responsible way that protects user privacy, yet it’s essential when you consider how privacy violations can impact a company’s public image. Especially as this technology matures and becomes more pervasive in our daily lives, it’s essential to follow AI laws as they’re passed and develop more specific AI use best practices that align with your organization’s culture and customers’ privacy expectations.
For additional tips related to cybersecurity, risk management, and ethical AI use when it comes to generative AI, check out these best practice guides:
- Generative AI Ethics: Concerns and Possible Solutions
- Generative AI and Cybersecurity: Ultimate Guide
- Risks of Generative AI: 6 Risk Management Tips
The post AI and Privacy Issues: Challenges, Solutions, and Best Practices appeared first on eWEEK.
Read more here:: www.eweek.com/rss.xml